Document Exploitation
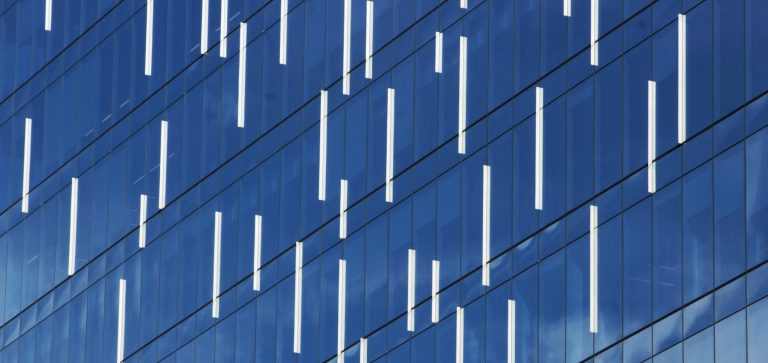
VALUE PROPOSITION
BlackSwan Technologies is reinventing enterprise software through Agile Intelligence for the Enterprise – a fusion of data, artificial intelligence, and cloud technologies that provides unparalleled business value. Our multi-tiered enterprise offerings include the award-winning platform-as-a-service, ELEMENT™, which enables organizations to build enterprise AI applications at scale for any domain quickly and at a fraction of the cost of alternatives. BlackSwan and its global partners also provide industry-proven applications that are ready-made and fully customisable for rapid ROI. These offerings are generating billions of dollars in economic value through digital transformation at renowned global brands. The private company maintains gravity centers in the UK, Europe, Israel, the US, and Sri Lanka.