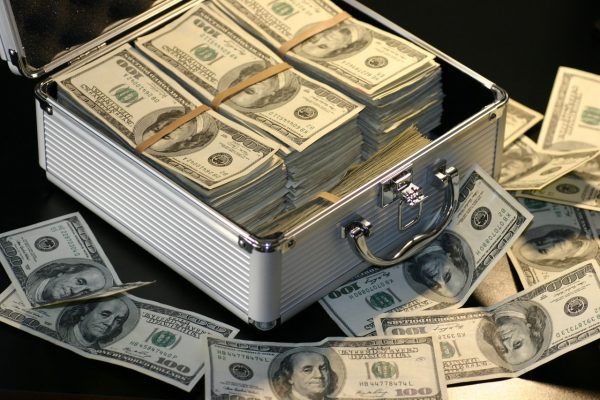
Nov. 16, 2021
How AI Can Be Used To Curb The Trillion Dollar Money Laundering Problem
By Harinder Singh Sudan @BlackSwan Technologies
Money laundering is a very sophisticated crime and we must be equally sophisticated — Janet Reno, while serving as Attorney General of the United States from 1993 to 2001
AML techniques are largely retrospective but AI enables new methods which can improve detection of money laundering
It has always been a battle for banks, governments and other organisations to ensure that the level of sophistication in detecting and thwarting money laundering attempts is up to the standard necessary.
The risk of not doing so can be staggering. The clandestine nature of financial crime makes it particularly difficult for entities to approximate the total sum of money that goes through the laundering cycle each year. The United Nations (UN) estimates the amount of money laundered globally to be 2-5% of all global GDP yearly, or $800 billion – $2 trillion.
The National Crime Agency (NCA) suggests that money laundering costs the UK economy around £24 billion every year and in 2015 the NCA estimated that at least £1.5 billion in criminal proceeds are laundered through banks and other money service businesses (MSBs), such as check cashiers and international remittances, in the U.K yearly. Due to increasingly stringent regulations, money launderers have looked elsewhere to funnel their illegally made profits and many have turned to MSBs as conduits through which they can deposit cash.
Many firms possess software capable of clearing upwards of $1 billion a month in transactions, or 12,500 transfers at an average of £80,000 per transfer.
Yet numerous firms, in both Europe and the U.S, have paid millions in fines due to failures associated with money-laundering controls and, as a result, have promised to further tighten their standards. As well as impacting the firms’ accounts, such fines can be hugely detrimental to the reputation associated with such institutions; if customers believe there is a greater risk involved with depositing funds and using the services of an MSB, they will inevitably decide to look elsewhere.
It should come as no surprise then, that one of the key ways in which companies have been attempting to address the money laundering challenge is through technology.
Overcoming the obstacles associated with obsolete technologies
While most firms recognise the pernicious impacts of money laundering schemes, many of them continue relying on obsolete systems and practices as a way of processing large volumes of sensitive data. Many procedures involve manual work by experts, hampering an organisation’s ability to effectively monitor transactions and identify potential money laundering activities.
The traditional monitoring capabilities of banks are largely retrospective, using rules and transaction-based methods, resulting in an inefficient system for alerting human investigators involved in detecting suspicious behaviour. Due to the complexity and time associated with enquiring into the origins of such alerts, false positives are far more prevalent than would ideally be the case. Traditional procedures also result in some banks needing to liaise with intermediaries in order to verify certain pieces of information, before making a regulatory disclosure. Many such banks rely on silos that have occurred naturally, sometimes through poor collection methods, adding friction to the AML process.
The majority of firms’ use sensitive AML trigger alerts, for instance a transaction occurring outside an account holder’s country of residence, generating an inordinately high number of false positives, each of which require human review as a means of assuaging related concerns. In the detection of money laundering, the scale of false positives presents an enormous burden. Due to frequent AML regulatory updates, institutions have to be aware of the necessary standards and adjust themselves accordingly.
The parameters used for transaction monitoring software and screening measures can make genuine customer behaviour appear suspicious. Such false positives are a drain on resources, and in some instances firms are unable to resolve AML alerts and are forced to correspond with a financial authority. Despite the burden associated with false positives, the majority of firms cannot afford to reduce their sensitivity to the measures which they use due to the potential financial or legal repercussions that could ensue. Nevertheless, false positives hinder the quality of customer experience, primarily due to the administrative work they produce, and disrupt firms’ abilities to address genuine money laundering incidents. Many of them are faced with the challenge of reducing the number of false positives without compromising the accuracy and effectiveness of AML transaction monitoring.
The unstructured process by which many organisations analyse vast amounts of data makes distinguishing false positives from genuine AML alerts particularly cumbersome. For the majority of institutions seeking to curtail money laundering activities, an equal regard should be given to both the quantity and quality of AML data, ensuring that the information concerned is sufficiently relevant, timely to the customer’s risk profile, and adequately structured. The complex process by which such data is processed is enormously burdensome for many institutions and can result in administrative ambiguity and hinder compliance’ teams ability to verify customer identities after an AML alert has been triggered.
AI can make the difference
The International Compliance Association (ICA) argues that, by comparison to traditional techniques, “AI has the ability to identify patterns of transactions, behaviours, and anomalies rapidly, allowing compliance professionals to better spend their time”.
Two modern techniques that incorporate AI to help firms gain a better idea of suspicious behaviours worth investigating are relationship and behaviour-based monitoring. These monitoring processes go beyond static rules to unearth hidden patterns and relationships between all entities and counterparties involved in any given transaction.
Relationship-based monitoring processes use visual link analysis to ingest and analyse large volumes of customer and transactional data, taking into account transaction history, ultimate beneficial ownership (UBO) and addresses. This helps to determine obvious and more complex connections between the entities and counterparties involved, and ultimately enables institutions to identify complex money laundering and terrorist financing networks more effectively.
Meanwhile, behaviour-based systems use machine learning algorithms to analyse customer and counterparty profiles and transactional data. This enables a firm to distinguish between normal and abnormal behaviour, uncovering hidden patterns that could be related to money laundering.
As these new techniques consider more complex factors than rule-based monitoring, criminals find it harder to circumvent these systems. In addition, machine learning enables these processes to evolve and keep up with new sophisticated methods that criminals are using. A combination of all three types of monitoring would provide banks and other organisations with the most robust and effective counter to attempts at money laundering and terrorist financing, and ultimately help enterprises to improve the management of risks.
With sophisticated techniques on the rise and regulatory procedures becoming more stringent, organisations need to reevaluate the risks associated with relying upon static traditional approaches. A greater number of financial institutions should be prepared to harness the benefits of AI and machine learning as a way of curtailing the trillion dollar money laundering problem.
BlackSwan’s Approach
BlackSwan’s ELEMENT of Compliance™ solution features leading-edge AML capabilities. Highlights include:
- End to End AML Solution incorporates data acquisition, rules engine, pattern diagnostics, alert generation optimisation, alert management, workflow and case management.
- Unified entity, fact and relationship tracking: Enable the enrichment and visualisation of entities, transactions, direct relationships, non-obvious relationships and networks via revolutionary Knowledge Graph technology.
- Continual insight improvement. Compliance effectiveness depends on the quality of insights presented by the system. Structured and unstructured machine learning generate powerful insights that enable analysts to quickly identify risks and repeated patterns – allowing faster and more informed decision making.
Harinder Singh Sudan is a Senior Vice President of the Financial Intelligence Unit at BlackSwan Technologies. He has close to 20 years industry experience in banking and financial services and leads BlackSwan Technologies’ FIU practice globally.
Learn more about ELEMENT of Compliance here or follow us on Twitter and LinkedIn