Predicting Class Action Lawsuit Profitability
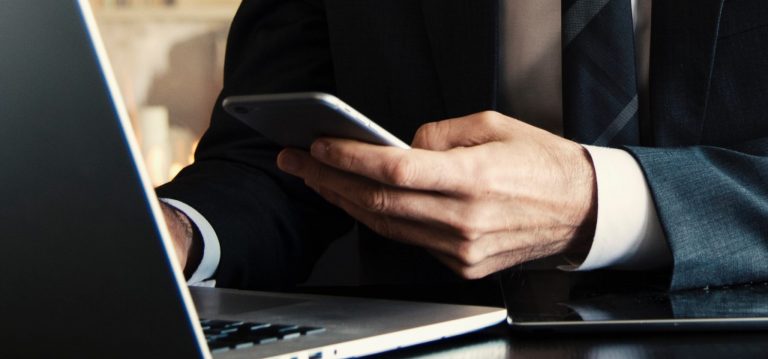
VALUE PROPOSITION
The firm sought an analytically-based approach to class action lawsuit success and profitability forecasting
Legal experts struggled to identify and monitor all available information sources and incorporate all influential factors
Reliance on human judgement resulted in subjective decision-making and sub-optimal effort allocation
BlackSwan Technologies is reinventing enterprise software through Agile Intelligence for the Enterprise – a fusion of data, artificial intelligence, and cloud technologies that provides unparalleled business value. Our multi-tiered enterprise offerings include the award-winning platform-as-a-service, ELEMENT™, which enables organizations to build enterprise AI applications at scale for any domain quickly and at a fraction of the cost of alternatives. BlackSwan and its global partners also provide industry-proven applications that are ready-made and fully customisable for rapid ROI. These offerings are generating billions of dollars in economic value through digital transformation at renowned global brands. The private company maintains gravity centers in the UK, Europe, Israel, the US, and Sri Lanka.